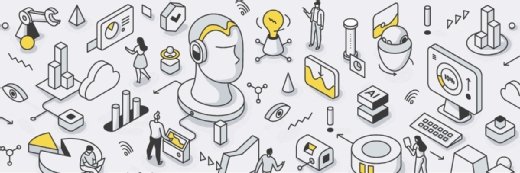
How AI is radically changing business process management
Deploying AI's discovery and automation capabilities in BPM powers advancements in front-office processes, process data analysis, business process mapping and process modeling.
Business process management has had a long history helping enterprises with their process engineering efforts and digital transformation initiatives. Now, BPM is getting a big boost from AI.
"AI technology is rapidly advancing, making it possible to develop more sophisticated and effective AI-powered process discovery and automation solutions," said Jeff Springer, principal consultant at data and analytics consultancy DAS42. Many of these advancements, he added, are due to the increasing availability of data from many sources such as enterprise systems, sensors and social media, resulting in AI deployments on a larger scale. The development of deep learning algorithms, for example, enables AI systems to learn from data and identify patterns that would be difficult or impossible for humans to identify.
How is AI transforming BPM?
AI-enabled deployments are finding numerous applications in BPM, ranging from improving front-office processes to analyzing process data to mapping business processes to exploiting generative AI process modeling capabilities.
Front-office processes
AI deployments in front-office processes is driving sales, increasing customer satisfaction and improving employee engagements, said Brian Steele, vice president of product management at call center intelligence platform provider Gryphon. In contact centers, for example, AI in business process management is enriching customer interactions, lowering call wait times, personalizing recommendations, and providing real-time sales assistance.
Process mining
Process mining is a key enabler of BPM, helping businesses discover opportunities to improve processes, create value and lower costs. "AI helps to make process mining much faster and easier to use, and inversely, process mining makes the data that the AI [system] is being trained on much more intelligent, unlocking its true power," explained Chris Monkman, vice president of product management, AI and knowledge at business process SaaS provider Celonis. But when it comes to training large language models (LLMs) and generative AI's struggle with hallucinations, innovations in process intelligence will require improvements in real-time structured data and semantic knowledge.
Object-centric process mining
Celonis and RWTH Aachen University are combining AI with object-centric process mining -- which represents real objects and events in a process -- to better understand and control business processes. When a real object like a shipping order or invoice moves through the business process, for example, AI can continuously update expected delivery times, send alerts in the event of delays and even take action to fix the problem.
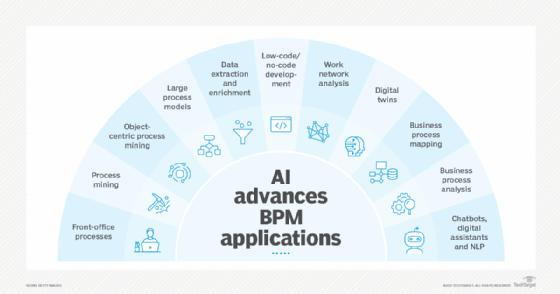
Large process models
Process management company SAP Signavio is using labeled data in LLMs to train what it calls large process models (LPMs) to analyze process data more accurately. SAP and academic researchers released the SAP Signavio Academic Models LPM data set, a collection of hundreds of thousands of business models, mainly in Business Process Modeling Notation. LPMs could be deployed in many use cases like best practice recommendations, process analytics, content creation and process data augmentation, according to Dee Houchen, head of global market impact at SAP Signavio.
Data extraction and enrichment
Optical character recognition software provider ABBYY is exploring ways AI technologies can extract more data from customer documents and correspondence to accelerate decision-making in enrollment, funding and approval processes, according to Bruce Orcutt, senior vice president of product marketing at ABBYY. AI could also be used to enrich data insights and improve process outcomes. "Data is king," Orcutt said, "but AI helps to make sense and bring context and meaning to all the data in an impactful way to the business."
Low-code/no-code development
Low-code and no-code tools are traditionally combined with BPM analysis tools to help streamline business reengineering efforts. AI is enabling more low-code/no-code development using GitHub Copilot capabilities, said John King, partner, business processes at Lotis Blue Consulting. This capability can promote the decentralization of application development and promises faster change velocity and more A/B testing types of deployments to meet customer needs. Businesses can also develop and support applications that automate critical business processes with only infrastructure and platform support from IT.
Work network analysis
Network analysis uses graph theory to understand the structure and function of complex systems. These same concepts, King surmised, could extend to the businesses through work network analysis that processes artifacts from meetings, phone calls, instant messages and emails. AI can identify and compare patterns of behavior and collaboration against the company's expectations and best practices to improve productivity where needed.
Digital twins
Digital twins are working models of physical environments and complex processes tied to the real world through a digital thread. AI can help transform raw data from sensors and workflows into more relevant digital twins. AI can also be applied to these models to provide different scenarios and decision analysis, King added. "This will save time and money," he reasoned, "and allow firms to model rare or prospective events before they occur, understand the impact of the event in a safe but also objective environment, and allow contingencies to be developed ahead of time."
Business process mapping
AI and machine learning models are already being used to automatically map out business processes and identify opportunities for improvement and automation, DAS42's Springer said. One manufacturing company, he noted, is using an AI system to monitor its production line in real time, identify potential bottlenecks and other issues, and recommend corrective actions to operators, which has resulted in a 10% increase in production output.
Business process analysis
Business process analysis traditionally has been done manually by process experts. AI in BPM could accelerate the outcomes of business process analysis for tasks involving modeling, collaboration, process mining, and risk management and compliance, said Stephen Ross, head of business development, Americas, at cybersecurity consultancy S-RM.
Chatbots, virtual assistants and NLP
Although chatbots and virtual assistants in one form or another have been around for nearly 60 years, their business value has only been realized in the past decade. Powered by generative AI, natural language processing (NLP) opens new business opportunities for chatbots and virtual assistants to be integrated into BPM systems to handle inquiries, guide employees through processes and improve customer interactions. NLP is also better at analyzing unstructured data sources such as customer feedback and social media posts to extract valuable insights.
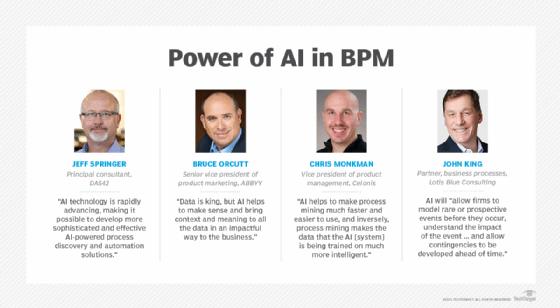
Benefits of AI in BPM
Citing the contact center as an example, Gryphon's Steele said AI in BPM can be used to discover opportunities for process optimization, increase efficiency, reduce costs and create value in the following ways:
- Identify and automate repetitive tasks to free up call agents to instead focus on more complex tasks and improve customer satisfaction.
- Route customers to the right agent or department to reduce call wait times and ensure customers receive the best possible service.
- Provide real-time assistance to agents to resolve customer service issues more quickly and efficiently.
- Analyze data to identify customer sentiment, trends and patterns leading to improvements in the customer experience.
Challenges of AI in business process management
Along with the benefits of deploying AI in BPM applications come the challenges, risks and ethical concerns, including the following:
- Lack of a holistic overview. There's no consensus on how generative AI can more broadly facilitate BPM.
- Generative AI's weaknesses. Concerns about accuracy, bias, repeatability, data privacy and hallucinations surrounding LLMs need to be solved by vendors homogeneously.
- Data quality. Data used to train and operate AI systems must be clean, accurate and complete.
- New data risks. Greater scrutiny needs to be applied to siloing AI within the organization and understanding where organizational data lives, what it consists of and how it's used.
- Lack of skilled workers. AI and BPM require specialized skills and knowledge, which will command additional investments in specialized training or hiring employees with the necessary skills.
- Fear of job displacement. Many organizations want generative AI and automation technology to work in lockstep, so they need to keep employees in the loop and at the center of the transformation.
- Ethical issues. Transparency, accountability and responsible use as well as potential biases and hallucinations are just some of the ethical considerations when applying AI to BPM.
George Lawton is a journalist based in London. Over the last 30 years he has written more than 3,000 stories about computers, communications, knowledge management, business, health and other areas that interest him.